Andrew is Co-I on the Sevilleta Long-Term Ecological Research proposal, led by PI Prof. Jenn Rudgers at the University of New Mexico, which has recently been awarded funding by the National Science Foundation. Andrew and Jacob are actively involved in the MVE (Mean x Variance Experiment), with 72 phenocams tracking daily changes in canopy greenness across 4 different biomes. You can read the UNM press release here.
Meet new PhenoCam team member Devin Jay San Nicolas
SICCS junior Devin Jay San Nicolas joined the PhenoCam project team at the end of the Fall 2024 semester. Born in Guam, Devin Jay has lived in Phoenix since 2016. In 2022, he enrolled in the BS in Computer Science program at NAU, and he expects to graduate in 2026. Devin Jay brings skills related to debugging and software engineering to the PhenoCam team. His ultimate career goal is game development. Outside of work and school he likes to dive into his own personal projects: writing game scripts, designing enemies and levels, and implementing gameplay mechanics. When he’s not doing that, however, Devin Jay likes to watch movies and TV shows with his family and friends. He is also fond of large snakes!
Welcome aboard, Devin Jay!
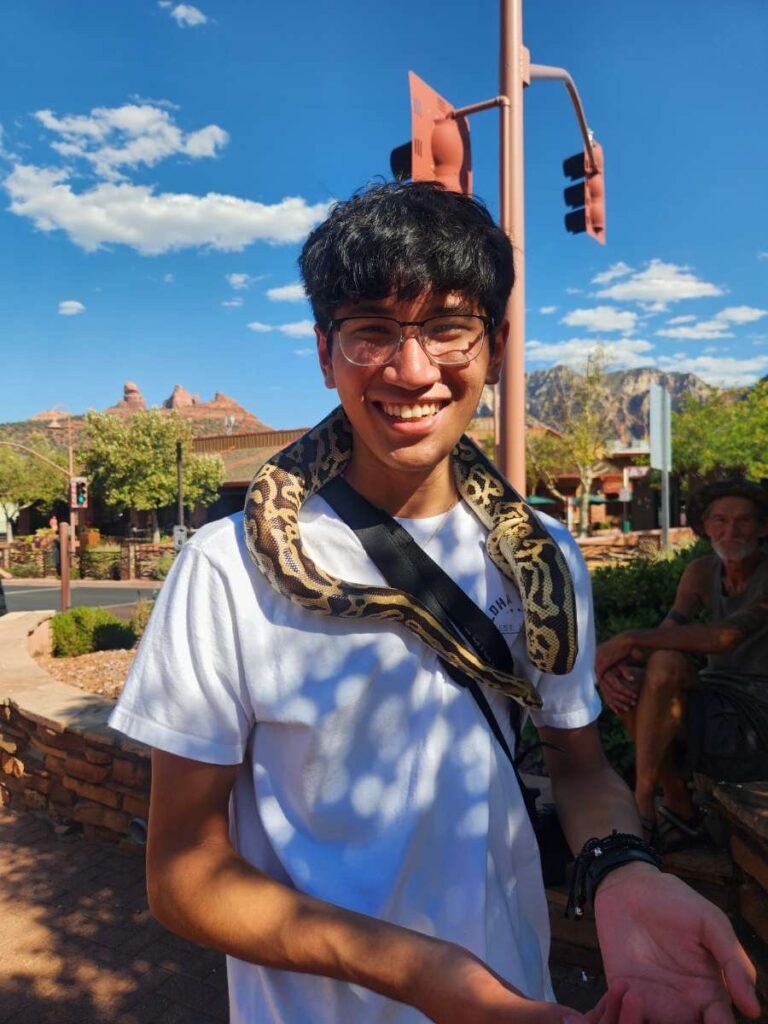
Paper on NSC reserves published in New Phytologist
Former postdoc Drew Peltier’s paper, “Decades-old carbon reserves are widespread among tree species, constrained only by sapwood longevity,” which uses a model-based approach to investigate how the distribution, mixing, and turnover of reserves vary across trees and species, has been just been published. Using data from 9 different species, Drew’s analysis shows that deep mixing and rapid turnover indicate that most photosynthate is rapidly metabolized. But, results also indicate that ecological variation in reserve ages is enormous, perhaps driven by stress and disturbance. Across all species, maximum reserve ages appear primarily constrained by sapwood longevity, and thus old reserves are probably widespread.
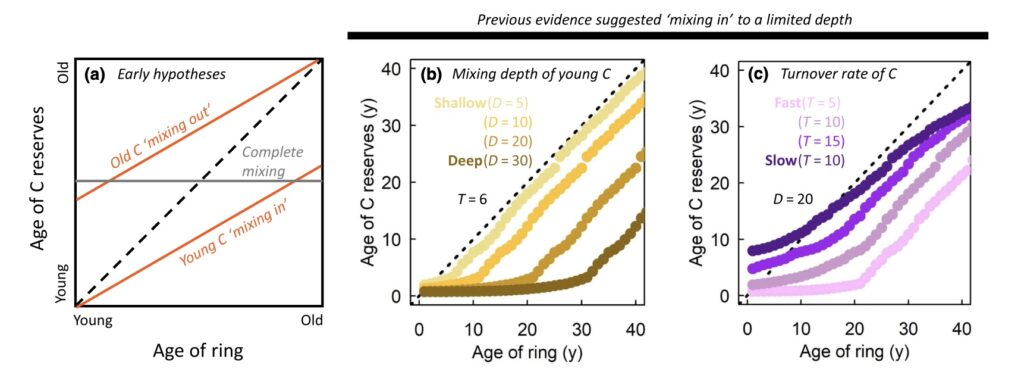
NEON Data Products paper out in Ecosphere
Andrew was a member of the first cohort of NEON Ambassadors, a diverse group of researchers and educators tasked with promoting the use of NEON data and growing the NEON user community. Together with fellow Ambassadors Jeff Atkins (USDA FS), Kelly Aho (Michigan State), Andrew Elmore (UMCES), and Danica Lombardozzi (Colorado State), he organized a virtual workshop for early career scientists in the summer of 2023. The workshop’s objective was to come up with guidelines for best practices in creating, validating, and using data products derived from NEON data. The workshop resulted in a paper that has just been published in Ecosphere, “Recommendations for developing, documenting, and distributing data products derived from NEON data.” The organizing committee thanks NEON for sponsoring the Ambassador program and this workshop.
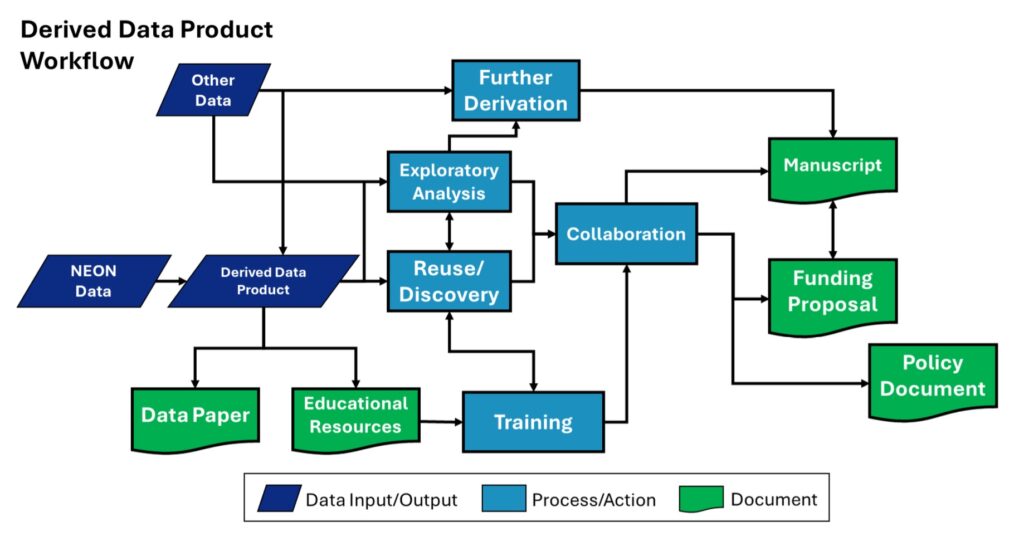
New machine learning paper using NEON data
Darby, Yujie, and Andrew are coauthors on a paper led by Jeffrey Uyekawa and Ben Lucas of NAU’s Department of Mathematics and Statistics in a special Landscape Ecology section of the journal Land. The study uses the Extreme Gradient Boosting machine learning model to model land-atmosphere CO2 fluxes, at 30 minute temporal resolution, across 44 NEON sites. In addition to standard k-fold cross-validation techniques, the study applies a “leave-one-site-out” (L1SO) approach to test predictions at a site which had not been used, in any way, to train the model. The results show strong potential for machine learning-based models to make more skillful predictions than state-of-the-art process-based models, being able to estimate the multi-year mean carbon balance to within an error ±50 gCm−2y−1 for 29 of 44 test sites. L1SO model performance was better when ecologically similar sites were included in the training data, and worse when there were no similar sites in the training data (e.g., more unique ecosystems in the Pacific Northwest, Florida, and Puerto Rico). Results also point to the enormous potential of machine learning to predict not only the long-term carbon balance of an unknown site, but even the inter-annual variation in that carbon balance. These results have significant implications for being able to accurately predict the carbon flux or gap-fill an extended outage at any AmeriFlux site, and for being able to make skillful predictions of ecosystem-scale carbon balance in support of natural climate solutions.
A companion paper on water fluxes is in preparation.
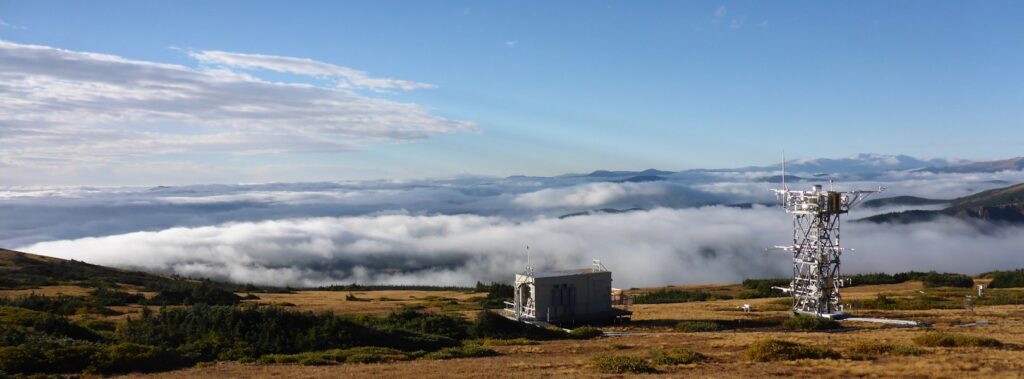
NEON tower at Niwot Ridge, Colorado (courtesy of NEON).
Lab contributes to new phenology book
Mark D. Schwartz, University of Wisconsin–Milwaukee, is well-known for his pioneering efforts—beginning in the 1980s—to bring the science of phenology into the modern era. He is also highly regarded as the editor of the book Phenology: An Integrative Environmental Science. The third edition of this book, published by Springer, was recently released. Across 644 pages, the book’s 27 chapters span a wide range of topics. You’ll see lab members’ names on several of those chapters:
• Perry and Andrew wrote the chapter on Mesic Temperate Deciduous Forest Phenology. Special congratulations to Perry on his first first-author publication!
• Oscar and Andrew wrote an updated version of the chapter on Near-Surface Sensor-Derived Phenology.
• Darby and Andrew contributed to the chapter on Phenology in Higher Education, which was led by long-time collaborator Theresa Crimmins of the USA-National Phenology Network.
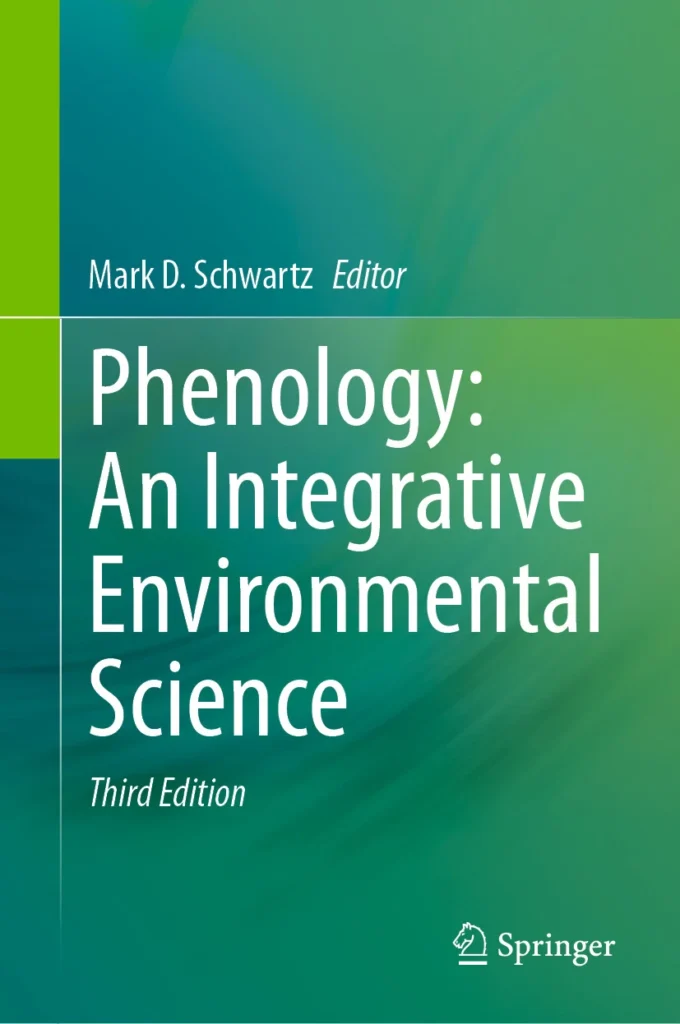